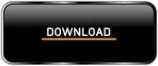
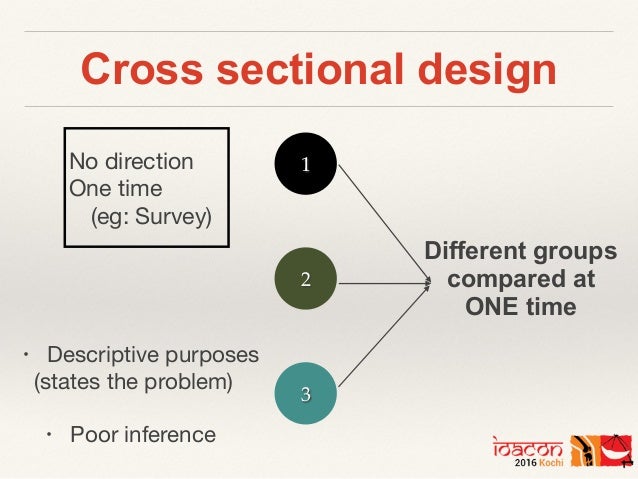
The lower ADCs were correlated with renal fibrosis in DN progression. Meanwhile, DWI provides information about tissue microarchitecture by evaluating the Brownian motion of water molecules ( 6– 8) tissues with restricted extracellular water content exhibit higher signal intensity in DWI and lower apparent diffusion coefficient (ADC) values ( 9). The higher R2* values suggest more hypoxia in the tissue. BOLD uses endogenous deoxyhemoglobin in the vessel as a contrast to detect MRI signal changes, which can reflect renal hypoxia ( 5). With the development of imaging technology, functional MRI (fMRI), including diffusion-weighted imaging (DWI) and blood oxygenation level–dependent MRI (BOLD), has been used to detect early renal function impairment and assess renal fibrosis ( 3, 4). Therefore, a more stable method is needed to reflect the different stages of renal function impairment. The eGFR may be lagged for the exact renal dysfunction in DN. However, renal function decline and subsequent renal fibrosis in DN are irreversible. Moreover, the eGFR increased at the early stage of DN for compensation, and it was easily neglected clinically ( 2). However, serum creatinine can be easily affected by medications or metabolism ( 1). At present, the assessment of renal function on DN mainly relies on the estimated glomerular filtration rate (eGFR). The outcome of DN is chronic progressive kidney damage and renal fibrosis. In recent decades, DN has become a major public health crisis and economic burden for dialysis to keep patients alive in China. Compared to the single T2WI sequence, mMRI-TA can improve the performance in assessing renal function.ĭiabetic nephropathy (DN) is a serious complication of diabetes that results in renal failure. Results: The mMRI-TA model achieved robust and excellent performance in classifying the sRI group, non-sRI group, and normal-RF group, with an AUC of 0.978 (95% confidence interval : 0.963, 0.993), 0.852 (95% CI: 0.798, 0.902), and 0.972 (95% CI: 0.995, 1.000), respectively, in the training cohort and 0.961 (95% CI: 0.853, 1.000), 0.809 (95% CI: 0.600, 0.980), and 0.850 (95% CI: 0.638, 0.988), respectively, in the testing cohort.ĭiscussion: The model built from multimodal MRI on DN outperformed other models in assessing renal function and fibrosis. The robust T2WI model was selected to construct a multimodal MRI model by combining the measured BOLD (blood oxygenation level-dependent) and diffusion-weighted imaging (DWI) values. The values of area under the curve (AUC) on receiver operating characteristic (ROC) curve analysis were used to assess their performance. Analysis of variance (ANOVA) and relief and recursive feature elimination (RFE) were applied to select the important features and then support vector machine (SVM), logistic regression (LR), and random forest (RF) algorithms were used for the model construction. Based on the largest coronal image of T2WI, the speeded up robust features (SURF) algorithm was used for texture feature extraction. According to the estimated glomerular filtration rate (eGFR), patients were assigned into the normal renal function (normal-RF) group, the non-severe renal function impairment (non-sRI) group, and the severe renal function impairment (sRI) group. Methods: For this retrospective study, 70 patients (between 1 January 2013 and 1 January 2020) were included and randomly assigned to the training cohort ( n1 = 49) and the testing cohort ( n2 = 21). We aimed to determine the possible practicability of machine learning (ML)-based multimodal MRI texture analysis (mMRI-TA) for assessing renal function in DN. A more stable method is needed to reflect the different stages of renal function impairment. Introduction: Diabetic nephropathy (DN) has become a major public health burden in China. 6Department of Nuclear Medicine, ZhuJiang Hospital of Southern Medical University, Guangzhou, Guangdong, China.5School of Medicine, South China University of Technology, Guangzhou, Guangdong, China.4Division of Nephrology, Guangdong Academy of Medical Sciences/Guangdong Provincial People’s Hospital, Guangzhou, Guangdong, China.3Department of Radiology, Guandong Academy of Medical Sciences/Guangdong Provincial People’s Hospital, Guangzhou, Guangdong, China.2Department of Radiology, Huizhou Central People’s Hospital, Huizhou, Guangdong, China.1Department of Radiology, The First Affiliated Hospital of Jinan University, Guangzhou, Guangdong, China.Wenbo Chen 1,2†, Lu Zhang 1, Guanhui Cai 2†, Bin Zhang 1, Zhouyang Lian 3, Jing Li 4†, Wenjian Wang 4,5*, Yuxian Zhang 6* and Xiaokai Mo 1*
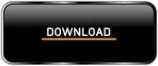